Running Local AI Models: Why Centralized Processing is No Longer Enough
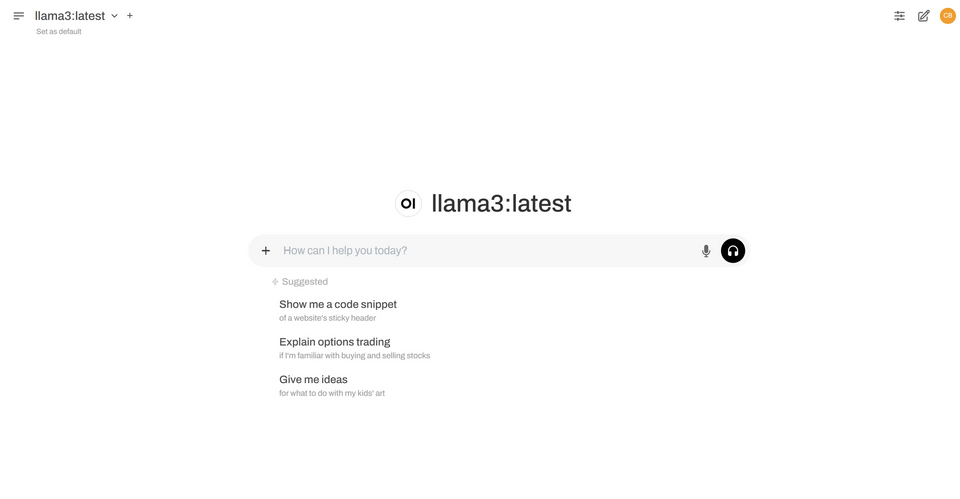
In today's data-driven world, Artificial Intelligence (AI) has become an integral part of many industries and applications. As the demand for AI-powered solutions continues to grow, so does the need for efficient processing of vast amounts of data. While centralized cloud-based processing has been the norm, there's a growing trend towards running local AI models on devices or edge nodes. In this blog post, we'll explore the benefits of running local AI models and why it's an essential step forward in the development of AI applications.
“Generative AI is the most powerful tool for creativity that has ever been created.It has the potential to unleash a new era of human innovation.” ~Elon Musk.
What is Running Local AI Models?
Running local AI models means processing data locally on a device or edge node, rather than sending it to a centralized cloud-based server for processing. This approach enables AI algorithms to analyze data in real-time, without relying on internet connectivity or latency-prone cloud computing.
Benefits of Running Local AI Models:
- Faster Processing Times : By processing data locally, AI models can respond quickly and accurately, making them ideal for applications that require instant decision-making.
- Improved Data Security : Running local AI models eliminates the need to transmit sensitive data over the internet, reducing the risk of data breaches and cyber attacks.
- Reduced Latency : Local processing reduces latency, ensuring that AI models can respond quickly and accurately, even in situations where internet connectivity is unreliable or non-existent.
- Increased Autonomy : Running local AI models enables devices to operate independently, without relying on centralized servers, making them ideal for applications such as self-driving cars, drones, and robots.
- Enhanced Data Privacy : By processing data locally, AI models can comply with increasingly stringent data privacy regulations, such as GDPR and CCPA.
- Increased Efficiency : Running local AI models reduces the need for constant internet connectivity, making it ideal for applications where internet connectivity is unreliable or expensive.
- Improved Performance : Local processing enables AI models to take advantage of device-specific hardware, such as GPUs and CPUs, resulting in improved performance and accuracy.
Real-World Applications:
- Self-Driving Cars : Running local AI models on vehicles enables them to make decisions quickly and accurately, without relying on internet connectivity.
- Industrial Automation : Local processing enables machines to operate independently, reducing the need for centralized control and increasing efficiency.
- Healthcare : Running local AI models on medical devices can enable real-time diagnosis and treatment, improving patient outcomes.
- Smart Homes : Local processing enables smart home devices to respond quickly and accurately to user commands, making them ideal for applications such as voice assistants.
Conclusion:
Running local AI models is an essential step forward in the development of AI applications. By processing data locally, AI models can provide faster, more accurate, and more secure results, while also reducing latency and increasing autonomy. As the demand for AI-powered solutions continues to grow, we can expect to see a greater emphasis on running local AI models on devices or edge nodes. Whether it's self-driving cars, industrial automation, healthcare, or smart homes, running local AI models is an essential component of creating more efficient, effective, and secure AI applications.